Today’s sophisticated Voice Assistants could be easily mistaken for something straight out of Netflix’s Black Mirror TV series. Back in 2005, the best smartphone could simply speak the time in a thick ‘robot accent’.
Fast forward to 2019, we are now surrounded by highly-evolved voice assistants that can hold a decent conversation with you or even book a haircut appointment for you, by dialing a real person in flesh and blood. Here’s a video where Google CEO Sundar Pichai shows off their Assistant having a humanlike conversation with someone at a hair salon and a small restaurant.
Voice assistants have certainly come a long way, but have you wondered how they gained all this intelligence in a matter of just a little more than a decade?
The simple answer to this is data, and more specifically user-generated data sourced from the web. User Generated Content is defined as any type of content that has been created and put out on the public web by unpaid contributors. In order for robots to understand us better, the developers are feeding them with more and more of the content that internet users put out there in the form of blogs, tweets, forum posts, product and hotel reviews and what not!
What constitutes User Generated Content?
The internet is growing at a staggering pace and this pace is only increasing with each megabyte uploaded to the web. And user generated content aka UGC constitutes a huge chunk of the web data that’s being created.
User generated content often doesn’t have proper grammar and punctuation usage. In fact, it comes with too many variables such as different dialects, slang, double entendre, sarcasm and non-standard grammar usage. This is exactly what makes it a great data source for machines that are meant to assist humans by learning the way they speak naturally.
Natural Language Processing is what helps us tackle this problem and help make machines understand and even talk back to us. For those who are unfamiliar with the term, natural language processing is an extension of data science that uses computational techniques for analysing and synthesising natural language and speech. So now, let’s have a look at what user generated content is being used for training NLP systems.
Although UGC can be found everywhere on the web, a broad way to classify it would be as follows:
1. Reviews on Ecommerce and travel portals
Product reviews on ecommerce portals is a much sought after source of user generated content, owing to its abundance on the web. You can find millions of reviews for all kinds of products, which makes it a great source of UGC for building voice solutions that could understand products or items that us humans use and seek. For example, if Siri were to understand and respond to a query such as ‘What smartphone has the best battery life?’, it should know about battery life as a variable pertaining to smartphones. And this kind of knowledge comes from being trained with UGC.
2. Tweets
Tweets are not only a great source of user generated content but it also helps act as a predictor for various world events. According to Twitter’s own statistics, 500 million tweets are sent out each day and that’s 5,787 tweets every second. That’s a lot of study material for our robot buddies.
Sentiment analysis is another use case of tweet data that’s gaining popularity in the recent times, which again helps the machines better understand the context of natural human speech by taking cues from the sentiment expressed in these tweets. Tweets are often brief and short, which is usually how humans like to interact with their voice assistants. This makes it a very ideal source of training data for NLP.
3. Blogs
There are about 600 million blogs in the world as of 2019 and that’s 13.66% of the internet users with a blog. Blogs are not only a great source of UGC but also a relatively easier one to extract from the web, thanks to RSS feeds and schema markup. The comments section of blogs is yet another place to source user generated content.
4. Forums and Q&A sites
Community forums are places where long, in-depth conversations about pretty much everything under the sun happens online. People tend to use their natural language style while conversing in forums and that’s great for data miners looking to build NLP solutions. Another goldmine of user data is the question and answer style websites like Quora which have had a revival in recent times after the demise of Yahoo! Answers.
How is User Generated Content extracted from the web?
Sourcing user generated content from the web is mainly done with the help of web data extraction solutions. It is simply a way of automatically extracting publicly available data on the web by programmatic means. Depending on the exact nature of the data extraction requirements, scraping can be done by using open source libraries like Beautifulsoup for Python, DIY tools and for those who want to focus only on the application of the data, by partnering with a fully-managed service provider.
History of Voice Assistants
Although voice assistants might seem like a thing of recent times, the first ever voice assistant was displayed by IBM in the year 1962 at the Seattle World Fair and this device was called Shoebox. It was actually the size of a shoebox and could understand and speak 16 words and 0-9 digits in human recognizable voice.
However, from the below timeline, we can see that Apple’s Siri was the first of Voice Assistants as we know them now.
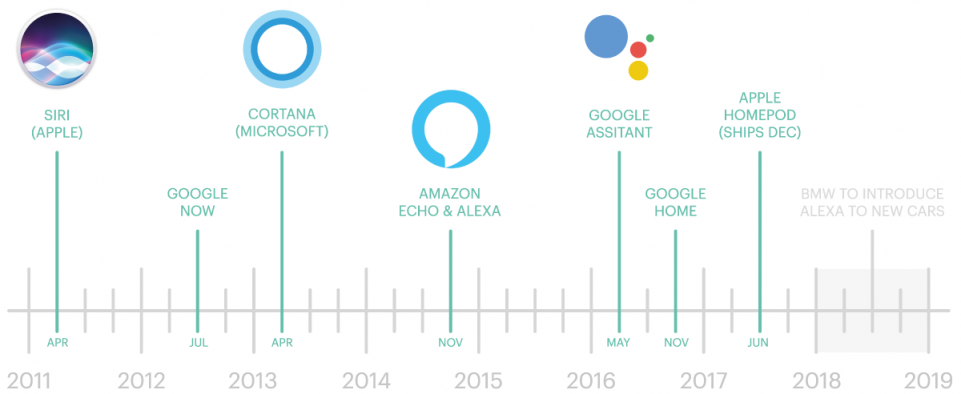
Voice Assistants: how do they work?
A voice assistant is basically a program with a human-side interface that can speak with a natural human tone. Under the hood, it generally consists of a Natural Language Processing system and API for fetching data from the web. The NLP system is the real brain of a Voice Assistant and is directly responsible for its ‘intelligence’. To put it simply, a voice assistant is as smart as the NLP algorithm allows it to be. And how do we make smart Voice Assistants smarter? Train them with more user generated content.
What does the future hold?
The advancements in data science as well as the emergence of better ways to access web data are making it possible for digital voice assistants grow sophisticated and become a part of our everyday lives. Voice has already established itself as the future of smartphone experience and this trend is here to stay.
If your business lacks the skills and expertise to adopt a voice strategy now, expect to find yourself left behind in the near future when voice takes over the world by storm. Voice brings to the table a unique opportunity for enabling deeper conversational experiences with customers which wouldn’t have been possible in the past. Now the question is, is your business voice ready yet?